Synthetic data opens up possibilities in the statistical field
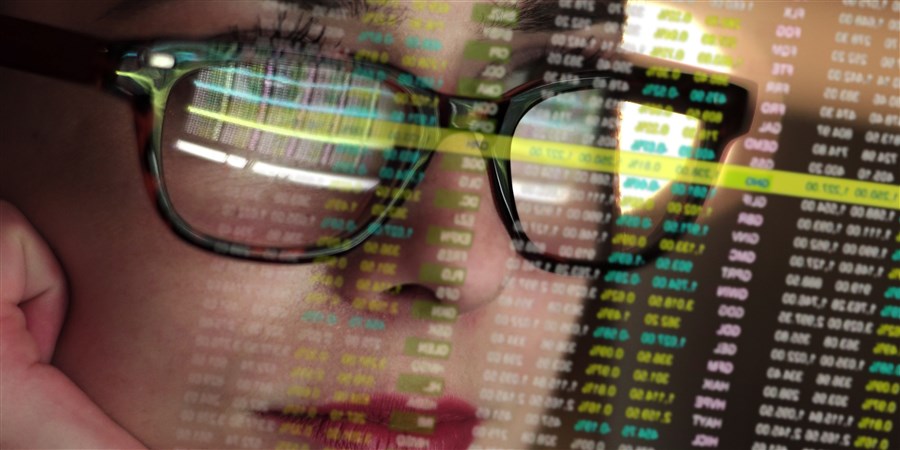
Synthetic data adds value
CBS holds a substantial amount of data involving personal information for which confidentiality has to be guaranteed. Although both the demand for data and the amount of data available continue to grow, there is still too little data exchange with the scientific world and private sector. From a business perspective, there is a need for improved data exchange methods to respond to increasingly stringent privacy regulations and the obstacles they present in terms of sharing data. Synthetic data can offer a means of solving this problem, always bearing in mind that legislation such as the General Data Protection Regulation (GDPR) also applies to the use of synthetic data. In addition to the benefits of synthetic data for testing new IT systems, CBS sees advantages in using those data for purposes such as simplifying data sharing with external partners. The need for further research and knowledge regarding certain aspects of synthetic data is being met through cooperation at both national and international levels.Challenge
Kate Burnett-Isaacs, Innovation Manager at Canada’s statistical institute, was appointed project leader for the UNECE handbook on synthetic data. How does she view the importance of these data? ’National statistical institutes prioritise access to data, transparency and openness,’ she explains. ‘The challenge lies in finding a safe and sustainable way to make it faster and easier to gain access to up-to-date, integrated data while at the same time maintaining data confidentiality. Synthetic data offer an opportunity to make it easier for users to analyse a wealth of more accurate and confidential data than historically available to the public.’Microsimulations
Synthetic data are not a new phenomenon. ‘But the introduction of more and more new methods and resources creates a need for a standard guide to their uses and risks. Our publication responds to the request of the High-Level Group for the Modernisation of Official Statistics, which sees the importance of such a guide in encouraging the use of synthetic data and stimulating the relevant discussion. Statistical institutes around the world face the same opportunities and the same challenges, so this is an ideal opportunity for international collaboration.’ Burnett-Isaacs’ colleague Steven Thomas explains that Statistics Canada already allows external partners to use synthetic data in certain situations: ‘Students who are interested in these data for training purposes, for example. The data are also valuable to researchers, both to help them prepare analyses and to confirm that those analyses will be feasible before they bring in the actual data. That said, synthetic data are most useful to the external researchers who work with microsimulations, which simulate real-life situations. Those researchers can gain a more detailed understanding of specific situations and conduct comprehensive analyses of the benefits and drawbacks of different scenarios.’
Testing algorithms
Christopher Jones, from the UNECE’s Statistical Division in Geneva, was closely involved with the project. He expects the use of synthetic data to increase significantly in the coming years. ‘Synthetic data can sometimes be as good as datasets generated by sampling from real data,’ he explains. ‘Bearing in mind that real data might themselves be collected as a result of sampling (for a survey), then you start to see its potential as a means of sharing insights in the form of microdata, fairly safely and accurately.’ However, he points out that one first has to overcome various problems, such as how to define the terms “safely” and “accurately”, given a particular (real) dataset and synthesis method. ‘Because of the trade-offs between the analytical accuracy of synthetic data and the risk of revealing confidential information, synthetic data are often limited in their use to testing of algorithms and new methods prior to their application on real data,’ he says. ‘Using synthetic data to directly produce analytical results, would result in a sacrifice of accuracy and/or safety.’Educational purposes
Manel Slokom works for CBS and CWI (National Research Institute for Mathematics and Computer Science, Ed.). She has studied synthetic data extensively, both for CBS and for her soon-to-be completed PhD at TU Delft, and she co-authored the UNECE handbook. ‘Synthetic data are data which resemble actual data but which are really fake or artificial because they are produced by machines,’ she explains. ‘CBS views synthetic data as data that are generated from computer simulations or algorithms, whilst preserving as much as possible of the analytical value that reflects the real world and minimising the risk of disclosing individual details. We currently use synthetic data within CBS for educational purposes and to test systems.’Advantages and disadvantages
In Slokom’s view, the challenge of using synthetic data is to see how it can protect privacy-sensitive information. ‘Secondly, these data can be used to reduce bias in datasets, because the data can be generated in such a way that they are not influenced by prejudices that could exist in the actual data. Slokom emphasises how important it is to determine the purpose for which the data will be used before starting the analysis, and of course there are also disadvantages associated with using synthetic data. ‘Synthetic data don’t reflect all the characteristics of real data, and it can be hard to guarantee accuracy. The use of these data can also lead to problems in interpretation, because it is not always clear how the data were generated. After all, behind every machine there’s a software developer. Those developers need to be able to understand and explain exactly what has gone into the synthetic data, and they need to document how the data were generated and what they can and can’t do.’Related items
- Article - What is synthetic data?
- CBS privacy regulations - Privacy
- Website - UNECE - Synthetic Data for Official Statistics - A Starter Guide