Misclassification bias in statistical learning
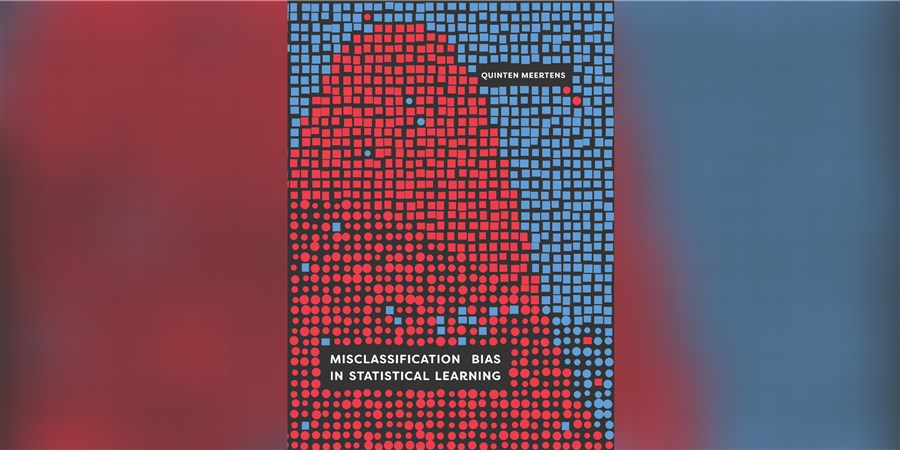
This thesis focuses on a specific group of statistical learning methods, namely classifiers. When the output of a classifier is aggregated, one obtains classifierbased statistics. If a classifier is not perfect, the resulting classifier-based statistics suffer from misclassification bias. To correct for that bias, a test set containing perfect information on the true classifications is required. A key challenge is selecting a correction method, in particular when dealing with time series that are non-stationary (i.e., that suffer from concept drift). The following open problem in the literature is raised: no solid theoretical analyses of methods correcting for misclassification bias in finite populations exist. Hence, the problem statement is formulated as follows: In what way can we reduce misclassification bias in statistical learning so that we obtain more accurate classifier-based statistics?
The conclusion of this thesis is that statistical learning methods can be used in the field of official statistics as long as misclassification bias is adequately corrected for. Our recommendation is to implement statistical learning methods (and the correction methods for misclassification bias discussed in this thesis) either to create newofficial statistics or to improve existing ones. Finally, we argue that domain experts are of vital importance to the successful implementation of statistical learning methods within official statistics.
Meertens, Q. A. (2021). Misclassification bias in statistical learning. Dissertation, University of Amsterdam, handle:11245.1/4b031bbd-5a46-4181-b0f1-52b38a3b63a6