COVID-19 hospitalizations: fostering decision-making in future pandemics
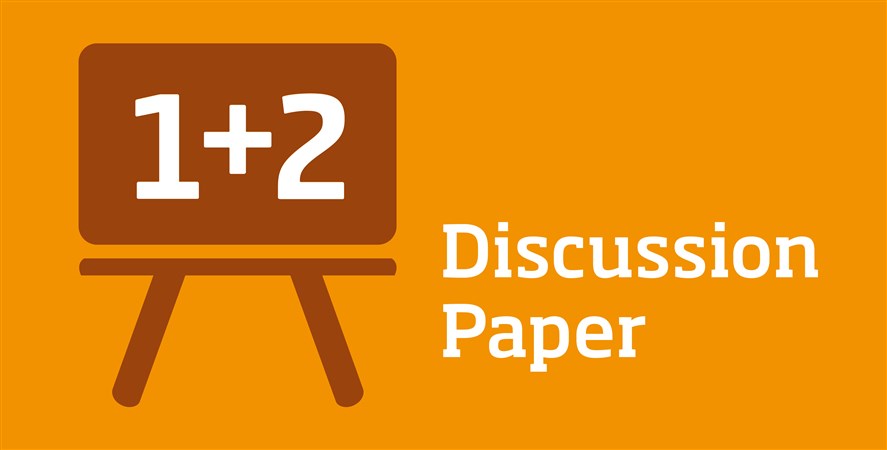
The fixed sensors provide data on pedestrian flows in metropolitan areas. The information from the mobile sensors is based on anonymized mobile phone data from apps like Google Maps. The WTP data informs about the coronavirus particles in wastewater. The weather data informs about temperature and humidity. The policy data provides daily policy measures that governments have implemented to address the pandemic.
The relationship between the indicators and the weekly COVID-19 hospitalizations is estimated using structural time series (STS) modeling. The STS models decompose the observed hospitalizations into a trend and a regression component. Each time-dependent regression coefficient describes how the relation between an auxiliary series and the hospitalizations evolves during the COVID-19 pandemic. Considered trend components are the local level, smooth trend, and local linear trend models as well as models with a time-invariant intercept. Relevant auxiliary variables for the regression components are selected with a step-forward variable selection method. Models are fitted with the Kalman Filter after expressing them in state space form.
The different model outcomes are extensively evaluated and discussed. Thus, besides informing on the potential relevance of indicators to predict COVID-19 hospitalizations, this paper aims to comprehensively compare the various models, their results, and their implications.
Our analysis reveals important relations between various indicators and COVID-19 hospitalizations, highlighting the importance of some specific indicators that could be used for modeling and predicting hospitalization during a pandemic. By comprehensively assessing the predictive power of different indicators and model specifications, we foster informed decision-making during pandemics, facilitating more effective public health responses.