The accuracy of estimators based on a binary classifier
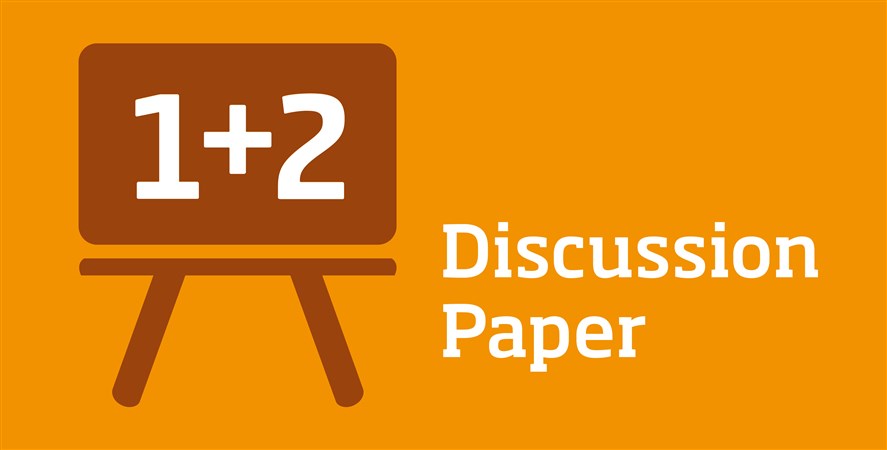
The accuracy of domain statistics is determined in part by the accuracy with which units are classified in their correct domains. With the increased use of administrative and other non-survey-based data sources in official statistics, as well as the development of automatic classifiers based on machine learning, it is important to account for the effect of classification errors on statistical accuracy.
Although bias and variance formulas for estimated domain totals and growth rates under classification errors have been derived before in the literature, these expressions are relatively complicated. Using those expressions, one cannot immediately obtain insight into the effect of classification errors on domain statistics in practice. The aim of the present paper is to provide some guidance, in the form of simple rules-of-thumb, on the behavior of domain statistics that may be expected in practice when classification errors occur. To keep matters as simple as possible, we restrict attention to the common case of counts with a binary classifier. We examine the accuracy of estimated proportions, differences of estimated proportions between two periods, and growth rates of estimated counts between two periods. The results are illustrated using a real text mining application on the prevalence of cybercrime in the Netherlands.
Downloads
- Paper - Classification errors binary